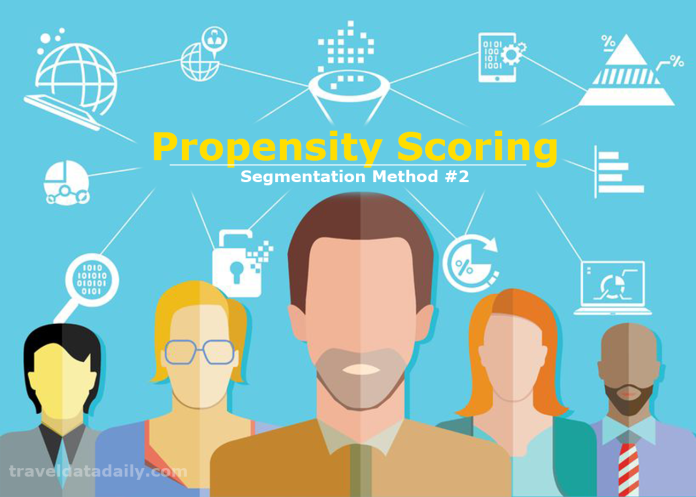
Audience Fragmentation, also known as behavioural scoring or propensity scoring, is a popular method of descriptive analytics which aims to calculate the likelihood of a user taking a particular action.
In my first loyalty segmentation article, I wrote about how to segment frequent flyer loyalty members based on demographics, account profile data and status levels which they had obtained within the frequent flyer program.
Once the framework for a segmentation engine is established, chances are it’s beginning to more like a data storage, business intelligence machine, rather than a static member database. That is the idea! For loyalty programs, the member database should be driving all marketing activity and acting as the centre for all decision-making around what campaigns are displayed to which members at any given time.
Traditional segmentation by audience type.
Making Audience fragmentation work
Audience fragmentation becomes increasingly more powerful when understanding consumer behaviour, or why someone transacted with product A instead of product B.
As a sub-set of prescriptive and predictive analytics, propensity modelling, or scoring, is a powerful addition to the data intelligence toolkit in which to use for marketing purposes. When combined with traditional segmentation techniques (i.e., demographic), the result is greater accuracy than ever before in ensuring the right user receives the right message at the right time.
By stacking these audience fragmentation models on top of each other, your customer profile becomes highly granular and allows for intelligence such as:
- Why a customer takes one action over another
- The likelihood of the customer buying Product A, but not the upsell to Product C
- Customer #112 was going to buy Product A anyway, so target them with Product D from Campaign A instead.
- The negative: Which actions won’t this customer take
- Who are high-value customers, and what incentivization (if any) might be required to drive incremental revenue?
How does propensity scoring work?
At the core, propensity scoring, or behavioural scoring is assigning a number to each customer (in advance), based on the multitude of past decisions, machine learning predictions, and similar customer actions which have been learned in the past.
To uncover the score for each customer, we would apply many different machine learning algorithms to identify patterns and similarities between this user’s previous engagement/transactions, and other customers who have engaged in a similar manner. (Transfer learning is also extremely handy – but that’s an article for another day)
The output of machine learning techniques used to recognise patterns might look something similar to this, which shows a 70%+ classification accuracy. This may or may not be high enough depending on how much data you have collected to feed into your model, but as a general rule of thumb – 70% can be considered accurate enough for prediction.
A simple way to work with the output would be to assign a number from 0-100, keeping in mind other positive and negative influencing factors outside of the model (higher the number, the more likely they are to purchase).
Which customers to target?
Just because a customer has a high score, does not mean they should receive the campaign material. For example, if the customer has a propensity score of 100/100, the chances of them purchasing the product are, statistically, extremely high. Chances are, it’s a simple case of the customer needing the product, rather than your ability to drive incremental new revenue. The user probably won’t require any additional incentivization to transact, and therefore a different message can be displayed to these users than the greater member base who will need a little nudge to help facilitate a transaction.
Understanding there is no single approach to data-driven marketing is essential. Propensity scoring, along with demographic segmentation techniques, represents another methodology to help extract greater insight and intelligence from your member database.
When the Audience fragmentation increases, it allows marketers to drill down on the core target market and focus the attention without wasting resources on groups of customers who are not likely to show purchase intent.
By targeting customers more effectively, it allows for the purchasing of digital media more effectively, as mentioned in by Reza Behnam.
Ultimately, the more you can learn from how and why customers interact with your brand, the more powerful and rich your analytics will become, thus driving greater revenue and increased enterprise value for your organisation.